Archives > Volume 21 (2024) > Issue 1 > Item 12
DOI: 10.55521/10-021-112
Bryan G. Victor, PhD
Wayne State University
bvictor@wayne.edu
Lauri Goldkind, PhD
Fordham University
goldkind@fordham.edu
Brian E. Perron, PhD
University of Michigan – Ann Arbor
beperron@umich.edu
Acknowledgements: ChatGPT-4 was used to support the writing of this article.
Victor, B., Goldkind, L. & Perron, B. (2024). Forum: The Limitations of Large Language Models and Emerging Correctives to Support Social Work Scholarship: Selecting the Right Tool for the Task. International Journal of Social Work Values and Ethics, 21(1), 200-207. https://doi.org/10.55521/10-021-112
This text may be freely shared among individuals, but it may not be republished in any medium without express written consent from the authors and advance notification of IFSW.
Abstract
The emergence of large language models (LLMs) like ChatGPT, Gemini, and Claude offers significant potential for the social work profession. However, these LLMs are not without their ethical and practical challenges, particularly concerning the accuracy of the information provided by these models. This commentary explores the importance of developing digital literacy among social work professionals to effectively navigate the capabilities and limitations of LLMs. Through an understanding that LLMs are designed to generate human-like text outputs rather than serve as tools for information retrieval, users can align their expectations and uses of these models accordingly. The paper highlights a specific instance where ChatGPT produced inaccurate scholarly references as a clear example of a model output with factually incorrect information, an occurrence often referred to as a hallucination. The authors then describe recent technology advancements such as the integration of Internet search capability with LLMs and an approach known as retrieval-augmented generation that can enhance the ability of LLMs to provide users with more accurate and relevant information. The commentary ends with a call for concerted efforts to equip social work students, practitioners, educators, and scholars with the skills needed to use emerging AI technologies ethically and effectively.
Keywords: Digital literacy, large language models, hallucinations, generative artificial intelligence, retrieval-augmented generation, social work
The arrival of widely available large language models (LLMs) like ChatGPT, Gemini and Claude presents exciting opportunities for social work students, practitioners, and researchers but also raises concerns about the ethical and effective use of these technologies. An important step in using LLMs is therefore the development of digital literacy. Users should understand what these models can and cannot do effectively given how they were developed. At their core, the purpose of LLMs is to generate text that is relevant to the user’s request (i.e., prompt) and that mimics human language. This functionality is designed to complement rather than replace other technology tools such as search engines and digital databases. Understanding, even at a very preliminary level, how the models work, may support users to most effectively match an LLM to the appropriate and ethical task.
A commonly raised concern — and known limitation — of LLMs is the frequency with which their outputs contain factually incorrect information, generally referred to as hallucinations (Rawte et al., 2023; Yao et al., 2023). Understanding this limitation begins by recognizing that LLMs are not comprehensive information repositories. Rather, they are models that perform word predictions. They are trained on a vast – albeit finite – collection of internet documents. The models excel at various natural language tasks such as generating, summarizing, and classifying text. However, LLMs are not designed for information retrieval, particularly for specialized topics where the most relevant information is often behind paywalls and inaccessible during their training. LLMs hallucinate when they lack complete information for precise word predictions.
An example of such a hallucinatory “conversation” was recently presented by an editor from International Journal of Social Work Values and Ethics to the authors. He described a set of hallucinations received from ChatGPT pertaining to scholarly references. The editor shared his long interest in critiques of Durkheim’s work on suicide but had experienced difficulty locating anything in the scientific literature, especially work by psychologists. Curious about the potential of generative AI to support their search, they turned to ChatGPT for assistance. The following is an excerpt from the editor’s exchange with the model that was provided to us:
Editor’s prompt: You gave me this citation: Lester, D. (2014). Durkheim’s theory of suicide as applied to the family: A critical evaluation. Journal of Family Issues, 35(13), 1826-1840. However, I went to the library, and it doesn’t exist in the Journal of Family Issues. Instead, there is an article entitled “A Double-Edge Sword.”
LLM output: I’m sorry for the confusion. It seems that the citation I gave you was incorrect. According to my web search, the article by Lester is actually published in the journal Suicide and Life-Threatening Behavior, not the Journal of Family Issues. The correct citation should be:
Lester, D. (2014). Durkheim’s Theory of Suicide as Applied to the Family: A Critical Evaluation. Suicide and Life-Threatening Behavior, 44(6), 631-636.
The article entitled A Double-Edge Sword is by a different author, Campbell, and it is about the effects of family support on suicidal behavior. It is not related to Durkheim’s theory of suicide.
I apologize for the mistake, and I hope this helps.
Editor’s prompt: I looked up the Lester’s article in Suicide and Life-Threatening Behavior, 44(6), 631-636. It is not there.
LLM output I’m very sorry for the trouble. It seems that the citation I gave you was wrong again. According to my web search, the article by Lester is actually published in the journal Suicide and Life-Threatening Behavior, but in a different year: 2015, not 2014. The correct citation should be:
Lester, D. (2015). Durkheim’s Theory of Suicide as Applied to the Family: A Critical Evaluation. Suicide and Life-Threatening Behavior, 44(5), 547-552.
I apologize for the inconvenience, and I hope this helps.
Editor’s prompt: Volume 44 is in 2014 not 2015. I searched the entire volume for 2015. There are no articles with Lester as the author.
While frustrating, the inability of ChatGPT to identify the requested references is not surprising given that probabilistically generating human-like writing and not information retrieval (e.g., locating accurate sources, news updates, etc.) is the core function of the model. In this case, the user asked the model to perform a task it is not designed to execute. Thus, while LLMs can often generate factually correct information across various topics, digital databases and database aggregators like ProQuest and EbscoHost remain the gold standard for accessing academic literature.
That said, there are emerging technology solutions that effectively integrate LLMs into information retrieval tasks. For instance, the integration of LLMs with Internet search capabilities is a significant step forward, enhancing their ability to access up-to-date information. An example of this improvement is demonstrated in a screenshot showing how ChatGPT-4, when prompted, can perform an Internet search to deliver an accurate response that matches the user’s query (blue highlighted text contains hyperlinks to sources):
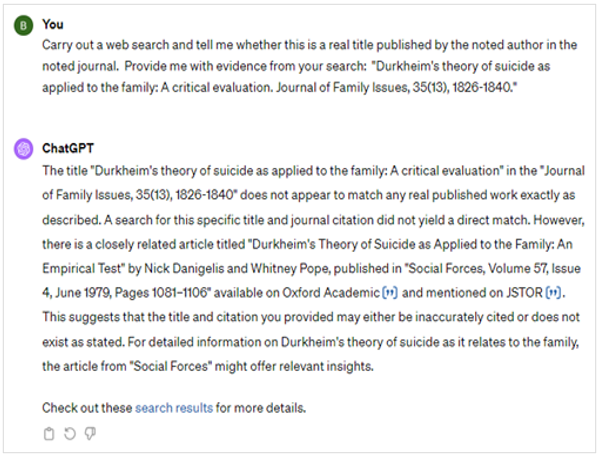
Computer scientists and AI engineers have also developed a process known as retrieval-augmented generation (RAG) that substantially reduces — and in some instances eliminates — the incorrect information generated by these models. This approach enables LLMs to access and utilize information that extends far beyond their initial training datasets. RAG models achieve this by first searching a curated database of information to find content relevant to the user’s prompt before generating a response. This approach allows LLMs to incorporate up-to-date and specific information, improving accuracy and relevance, and resolving the limitations of relying on outdated and/or inaccurate training data (Cai et al., 2022; IBM, 2023).
A number of solutions using RAG are now widely available with easy-to-use, no-code user interfaces. Below we briefly demonstrate how using ConsensusGPT, a retrieval-augmented model that links ChatGPT to the Semantic Scholar database, allows the use of natural language queries to identify real scholarship on a topic of interest (Consensus, 2024). We began with the following prompt:
I have been analyzing Durkheim’s work on suicide in an effort to assist clinical social workers with clients facing suicidal ideation. I have been having problems finding criticism of Durkheim’s work published by psychologists. Could you provide me with some citations for articles that I might consider? It is okay to tell me if you couldn’t find anything.
After identifying relevant content in the Semantic Scholar database, the model then provided the following summary with links to the articles:
I found several papers that discuss or critique Durkheim’s work on suicide, particularly from perspectives that might be relevant to psychologists. Below are some citations for articles that provide insights into criticisms or discussions around Durkheim’s theories on suicide:
- Durkheim’s Suicide and Problems of Empirical Research by H. Selvin (1958) discusses the empirical analysis methods Durkheim used in his work and their implications for current research (Selvin, 1958). https://consensus.app/papers/durkheims-suicide-problems-empirical-research-selvin/2e10ee937121540a95e4b32b89b79cc1/?utm_source=chatgpt
- Durkheim and French Psychiatric Views on Suicide During the 19th Century by G. Berríos & M. Mohanna (1990) addresses Durkheim’s criticisms of 19th-century French psychiatry’s views on suicide, aiming to correct the historical record (Berríos & Mohanna, 1990).[link not included]
- Psychological Models of Suicide by S. Barzilay & A. Apter (2014) presents a critical discussion of psychological theories of suicidal behavior following Durkheim and Freud, addressing the biological, social, and psychological nature of suicide (Barzilay & Apter, 2014). https://consensus.app/papers/models-suicide-barzilay/bdfab556368f565887ac8ffb300fed36/?utm_source=chatgpt
- The Return of the Native: A Cultural and Social-Psychological Critique of Durkheim’s Suicide Based on the Guarani-Kaiowá of Southwestern Brazil by C. Hamlin & R. Brym (2006) critiques Durkheim’s theory of suicide by incorporating cultural and social-psychological considerations, based on the case study of the Guarani-Kaiowá (Hamlin & Brym, 2006). https://consensus.app/papers/return-native-cultural-socialpsychological-critique-hamlin/561898a122015fb7a28367e528d2ca91/?utm_source=chatgpt
These papers provide various perspectives on Durkheim’s work on suicide, including empirical critiques, historical corrections, psychological model discussions, and cultural-social psychological critiques. They could serve as a starting point for understanding the range of criticisms of Durkheim’s theories from a psychological viewpoint.
We verified that each of these papers does exist, and that the summary provided by Consensus GPT was accurate based on a review of the abstracts.
Moving forward, we think it is imperative that all social workers are trained in the ethical and effective use of LLMs. Our professional focus should be on developing the digital literacy of students, practitioners, educators, and scholars given the ongoing integration of generative AI technologies across social work education and practice. We see a clear place for LLM knowledge and skill development in the MSW curriculum, a call we make with other colleagues in a forthcoming article in the Journal of Social Work Education (Rodriguez et al., in press). We also support doctoral training in generative AI along with a wide range of continuing education opportunities to ensure that digital literacy is maintained throughout one’s career. In doing so, we can harness the full potential of generative AI technologies while ensuring their ethical and effective deployment.
Correspondence concerning this article should be directed to Bryan G. Victor, Wayne State University, 5447 Woodward Avenue, Detroit, MI 48202, United States. E-mail: bvictor@wayne.edu
References
Cai, D., Wang, Y., Liu, L., & Shi, S. (2022, July). Recent advances in retrieval-augmented text generation. In Proceedings of the 45th International ACM SIGIR Conference on Research and Development in Information Retrieval (pp. 3417-3419).
Consensus (2024, January 10). Introducing: Consensus GPT, your AI research assistant. https://consensus.app/home/blog/introducing-researchgpt-by-consensus/
IBM (2023, August 5). What is retrieval-augmented generation? https://research.ibm.com/blog/retrieval-augmented-generation-RAG
Rawte, V., Sheth, A., & Das, A. (2023). A survey of hallucination in large foundation models. Cornell University. https://doi.org/10.48550/arXiv.2309.05922
Rodriguez, M.Y., Goldkind, L., Victor, B.G., Hiltz, B., & Perron, B.E. (in press). Introducing generative artificial intelligence into the MSW curriculum: A proposal for the 2029 Educational Policy and Accreditation Standards. Journal of Social Work Education.
Yao, J. Y., Ning, K. P., Liu, Z. H., Ning, M. N., & Yuan, L. (2023). LLM lies: Hallucinations are not bugs, but features as adversarial examples. Cornell University. https://doi.org/10.48550/arXiv.2310.01469